Have you ever imagined creating complex AI models without writing a single line of code? The rise of no-code AI model builders has transformed this seemingly futuristic notion into a tangible reality. For intermediate users eager to engage in AI innovation without delving into intricate coding skills, platforms like Cogniflow, Model.ai, and Akkio are game-changers.
Driven by a demand for accessibility and efficiency, no-code AI tools are empowering a new wave of developers to harness the power of AI. From crafting predictive models with Obviously AI to seamlessly integrating solutions into existing workflows, these tools are redefining how businesses approach AI application development. With over 15 innovative tools available to optimize operations, the landscape of AI has never been more inclusive or dynamic.
This article delves into expert tips designed to elevate your experience with no-code AI platforms. By exploring best practices and strategies across data management, deployment, and troubleshooting, readers will uncover the pathways to crafting successful AI model creations. Whether you’re looking to enhance your projects through machine learning no code or discover how to automate AI processes, this guide offers valuable insights tailored for your journey.
Key Takeaways
- No-code AI model builders like Cogniflow, Model.ai, and Akkio empower intermediate users to create and deploy AI models without coding expertise.
- Understanding the key features and capabilities of various no-code AI tools is crucial for selecting the right platform that aligns with business needs.
- Adopting no-code solutions enhances productivity by enabling users to automate AI processes and streamline AI application development efficiently.
- Data preparation and model training are critical for training models effectively; best practices should be followed to guarantee accuracy and continuous improvement.
- Deployment and integration strategies must focus on compatibility with existing systems to maximize the utilization of advanced AI features and leverage analytics for actionable insights.
- BurstyAI can significantly boost productivity through automation tools, SEO optimization, and seamless integration with platforms like Make and Zapier, enhancing overall workflow efficiency.
Understanding No-Code AI Platforms
Overview of Top Platforms: Cogniflow, Model.ai, Akkio
No-code AI model builders have revolutionized the way users approach AI application development. Prominent platforms such as Cogniflow, Model.ai, and Akkio stand out for their user-friendly interfaces and robust functionalities. These platforms allow individuals to construct, deploy, and integrate AI models seamlessly, paving the way for those with limited programming expertise to engage with AI technologies effectively.
Cogniflow emphasizes streamlining the model creation process, leveraging various data sources to automate AI processes. With its no-code environment, users can quickly build workflows that meet their specific needs.
Model.ai focuses on enhancing accessibility in AI, offering rich resources and capabilities that make it straightforward for intermediate users to create machine learning models without overwhelming complexity. Its community-driven features foster collaboration and shared knowledge among users.
Akkio is another notable player, recognized for its data automation features and emphasis on speed. Akkio helps users transition from raw data to predictive models in minimal time while offering extensive guides to facilitate the learning process.
Key Features and Capabilities
The key features of no-code AI platforms like those mentioned above include intuitive drag-and-drop interfaces, pre-built templates for common use cases, and easy integration with popular data sources and applications. Users can automate AI model creation by selecting from a library of algorithms that serve various business needs, which significantly reduces the barriers to entering AI.
Moreover, the analytics and visualization tools available in these platforms allow users to monitor performance and refine their models actively. This accessibility is vital for businesses looking to leverage AI innovation without needing extensive technical training.
Advantages of No-Code Solutions for Intermediate Users
For intermediate users, embracing no-code solutions presents numerous advantages. It allows individuals to maximize their productivity by focusing on problem-solving rather than on the intricacies of coding. The accessibility of machine learning no code platforms empowers users at any technical skill level to develop AI models that can optimize their operations.
No-code platforms also foster a culture of experimentation, as users can test and iterate on models rapidly without the fear of making coding errors. This environment encourages creativity and innovation, which can lead to more effective and tailored solutions.
Integrating AI Platforms with Existing Tools
Integration capabilities play a crucial role in maximizing the effectiveness of no-code AI platforms. Users can seamlessly connect these platforms with tools they already use—such as customer relationship management (CRM) systems, marketing automation tools, and data storage solutions. Integration enhances the AI model deployment process, ensuring that models are easily accessible and actionable.
Evaluating Platform Suitability for Your Needs
When considering the suitability of a no-code AI platform for specific needs, users should assess various factors, including the platform’s customization options, scalability, and the support available for troubleshooting. To make an informed decision, it can be beneficial to explore user reviews and case studies that highlight successful implementations.
In conclusion, by understanding the robust offerings of no-code AI platforms and their integration potential, intermediate users can leverage these tools to drive efficiency and innovation in their organizations.
Crafting the Perfect AI Model
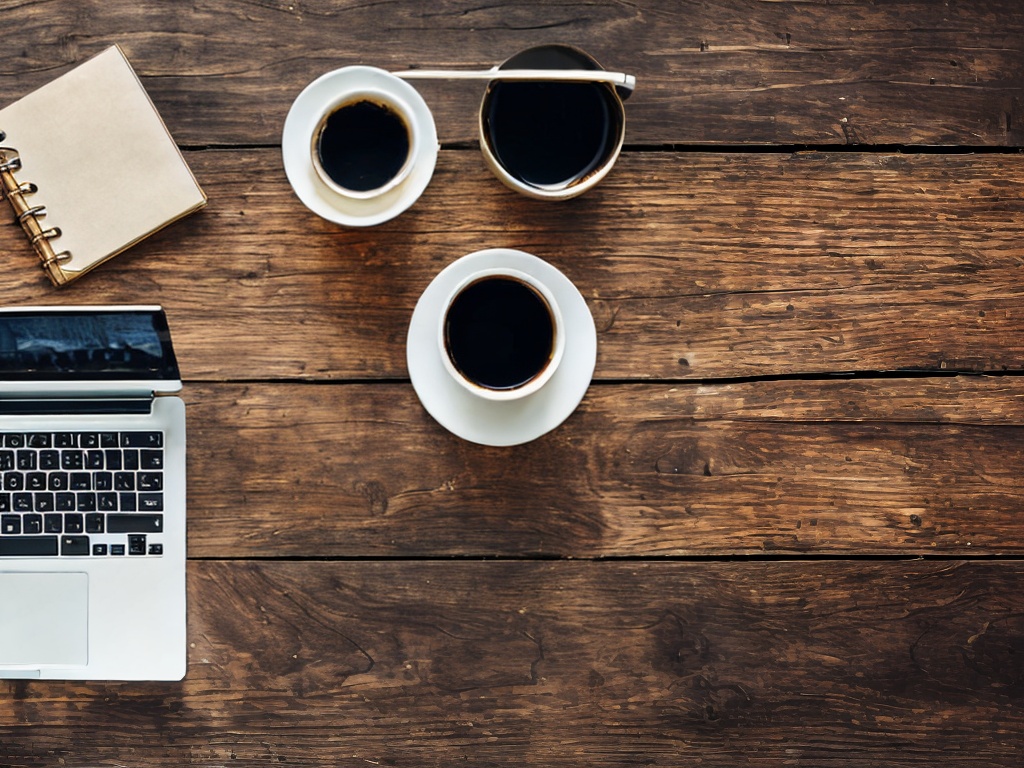
Data Preparation Best Practices
Effective data preparation is paramount in AI model creation. It sets the foundation for building robust models that deliver accurate insights. Intermediate users should prioritize cleaning their data to eliminate noise and inconsistencies. This can involve removing duplicates, handling missing values, and ensuring data types are correctly defined. Users may also want to standardize data formats, which can be pivotal for achieving consistent results across different AI applications.
Moreover, transforming raw data into a suitable format for no code AI tools is essential. Techniques such as normalization and encoding categorical variables are critical for enhancing model performance. Utilizing tools such as Cogniflow or Akkio can streamline this process, enabling users to efficiently manage their datasets in a user-friendly environment.
Finally, maintaining a clear documentation of the data preparation steps taken is beneficial. This not only aids in transparency but also assists in traceability, helping users to revisit and understand their decisions during the AI application development process.
Selecting the Right Model Type
Choosing the appropriate model is essential for effective AI model deployment. Users must consider the nature of their data and the specific problems they aim to solve. Common model types include regression models for continual outcomes and classification models for categorical outcomes. Each model carries its strengths and complications, and understanding these can significantly impact the success of the AI model.
Furthermore, leveraging machine learning no code platforms provides users with a plethora of model options without the need for extensive coding knowledge. For instance, platforms like Obviously AI and Model.ai offer a variety of pre-built models that can be customized for specific user needs. This flexibility allows intermediate users to experiment with different approaches to find the right fit for their project.
It is also beneficial to consider performance metrics when selecting models. Users should assess accuracy, precision, recall, and F1 score based on their expected outcomes. This initial step can save time and improve efficiency in later stages.
Training Models with Accuracy and Efficiency
Training models effectively requires a balance of efficiency and accuracy. Intermediate users should leverage techniques such as k-fold cross-validation, which allows them to maximize their training dataset while minimizing overfitting. This method helps fine-tune model parameters and ensures the model generalizes well to unseen data.
Using data automation tools in the training process can lead to significant time savings. For example, BurstyAI’s automation tools enable users to streamline repetitive processes, ensuring models are trained timely and efficiently. This capability is particularly useful for users who want to focus on optimizing model performance rather than getting bogged down with administrative tasks.
Additionally, monitoring model performance during training is critical. Users should implement performance tracking to assess how adjustments to parameters impact overall effectiveness, allowing for immediate corrections and enhanced learning outcomes.
Testing and Validation Techniques
Testing and validation are activities no less important than training. Employing robust techniques ensures that the AI models are not only functional but also reliable. Intermediate users are advised to use segmented datasets for testing—the holdout method is one of the simplest testing approaches, where the dataset is divided into training and testing parts.
After training, users should evaluate their models using unseen data to measure performance accurately. It is equally important to apply various validation techniques such as confusion matrices and AUC-ROC curves to obtain comprehensive insights into model performance and reliability.
If a model underperforms, users should not hesitate to revisit earlier stages, whether it be refining data or retraining models. This iterative approach fosters an atmosphere of continuous improvement and encourages growth within the overall process.
Iterating for Continuous Improvement
Continuous improvement is a key aspect of successful AI innovation. Intermediate users should embrace an iterative workflow, regularly revisiting and refining their AI model creation processes. This could involve forming a feedback loop where insights gathered from testing results drive further model adjustments.
Additionally, it is beneficial to stay updated with industry advancements and community AI models. Engaging with other AI practitioners through forums or collaborative platforms can provide fresh perspectives and inspiration for iterations, keeping their approach dynamic and adaptable.
Lastly, utilizing analytics tools that track performance over time can help identify trends and areas for improvement. By systematically analyzing the results, users can optimize their processes and ultimately enhance the effectiveness of their AI models.
Maximizing Platform Capabilities
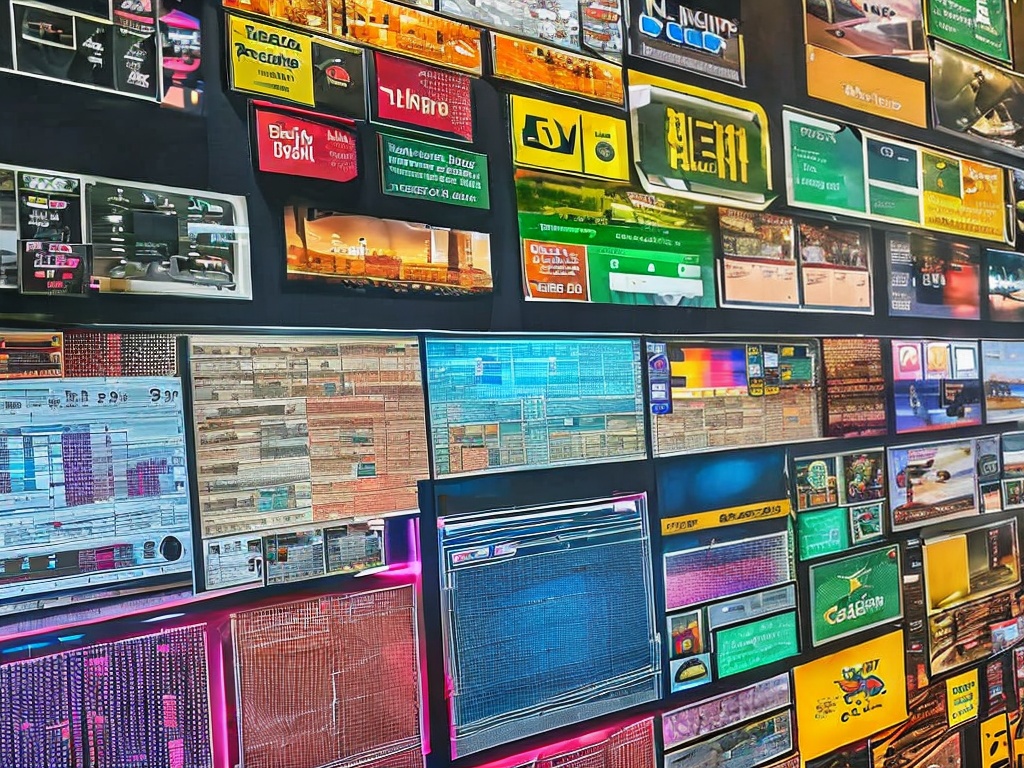
Advanced Features for Enhanced AI Models
When discussing the no code AI model builder, intermediate users should prioritize understanding the advanced features offered by various platforms. Tools such as Cogniflow, Model.ai, and Akkio provide functionalities that enhance model performance and efficiency. These features include pre-built templates, machine learning algorithms, and the option to manipulate datasets directly within the platform, enabling a smoother operation and integration into existing workflows.
By leveraging these advanced capabilities, users can quickly navigate through intricate data sets and deploy sophisticated AI solutions. Understanding specific features like autoML processes is vital as they simplify the construction of predictive models, thereby significantly reducing the time taken to bring a reliable model to the market.
Furthermore, users should seek tools that facilitate visualizations of data flow and model performance. Utilizing such features allows for better understanding and optimization of the AI model creation process.
Leveraging Automation in Model Building
Automation plays a crucial role in the success of AI application development. Intermediate users are encouraged to utilize automation tools incorporated within no-code AI tools to streamline their processes. This includes automating repetitive tasks, data cleaning, and model training, which can significantly enhance productivity.
Tools like Obviously AI emphasize speed, allowing the user to transform raw data into predictive models swiftly. Automating these processes not only saves time but also reduces errors that can occur during manual handling. This feature is particularly important in a fast-paced business environment where efficiency is paramount.
To maximize the potential of an AI platform, users should identify workflows that can be automated. Building an automation framework leads to consistent model training and deployment, allowing users to focus on developing innovative solutions rather than managing mundane tasks.
Customizing Models for Specific Use Cases
One of the most significant advantages of no code solutions is the ability to tailor models to meet specific business needs. Intermediate users should focus on how to customize their AI models effectively. Understanding the business objective and aligning the AI model to fulfill those objectives is key.
For instance, a business seeking to enhance customer engagement might customize their model to analyze customer behavior patterns. Platforms that support this customization often have functionality to adjust parameters and algorithms, allowing users to experiment with different approaches based on real-time data.
This level of customization not only ensures that the models are relevant to the business’s unique requirements but also contributes to higher success rates of project implementations. Thus, leveraging customization features available in no-code tools is essential for optimizing results.
Using Platform Analytics for Insights
Data-driven insights are paramount for decision-making in AI model deployment. Intermediate users should consistently utilize platform analytics to obtain comprehensive insights into model performance. Most no-code AI tools come equipped with built-in analytics features that can track key performance indicators (KPIs) of ML models.
By analyzing metrics such as model accuracy, prediction latency, and user engagement, individuals can identify strengths and weaknesses within their models. This information is vital for making data-backed decisions regarding model improvements or potential pivots in strategy.
Investing time in understanding analytical reports allows users to make continuous refinements, ensuring the AI models they deployed are tuned effectively for optimal outcomes. Furthermore, these analytics serve as a guide for future project developments.
Exploring Integration Possibilities
Finally, an exploration of integration possibilities is necessary for enhancing the effectiveness of AI initiatives. Intermediate users should look for business optimization tools that seamlessly connect their no-code AI models with other applications and platforms. Integration facilitates a more cohesive data ecosystem where information can be shared effortlessly.
For example, integrating AI models with customer relationship management (CRM) software can enhance marketing strategies by providing insights on customer interactions. Users should explore how their chosen AI platforms facilitate these integrations, as many offer plugins or APIs that significantly expand functional capabilities.
In conclusion, maximizing platform capabilities through advanced features, automation, customization, data analytics, and integration is critical for the effectiveness of no-code AI model builders. Intermediate users who actively engage with these functionalities will unlock greater potential within their AI initiatives and drive meaningful business outcomes.
Deployment and Integration Strategies
Steps to Seamless Deployment
Deploying an AI model requires a systematic approach to ensure that it operates correctly in a production environment. The first step involves understanding the AI model creation process and identifying the specific requirements for deployment. This could include the selection of appropriate deployment environments, such as cloud or on-premises solutions.
Next, proper data validation must be conducted. By examining the data used to train the model, users can ensure that it will function effectively when exposed to live data. In addition, conducting thorough testing helps in detecting any issues and ensures that the model achieves optimal performance.
Integration with existing systems is another critical phase. Intermediate users should plan out how the new model will interact with current applications and workflows. Tools such as Cogniflow or Akkio can aid in simplifying this process by offering user-friendly interfaces for integration tasks.
Lastly, a deployment plan should be established. This plan should detail each step, the roles of team members, and timelines to ensure a smooth transition from development to public use.
Ensuring Compatibility with Existing Systems
Compatibility is a key consideration when deploying AI models. Users must examine whether their AI platform supports the required APIs and data formats that existing systems utilize. This ensures seamless interaction between the new no-code AI model and pre-existing applications.
Utilizing modern APIs allows for more straightforward connectivity. Most no-code AI tools, including Model.ai, provide easy-to-use API integration features that align well with many mainstream business applications.
Another crucial aspect is performance measurement during integration. Users should continuously assess the feedback from the integrated systems to identify any mismatches or slowness in data transfer, which can hinder operations.
Real-World Integration Examples
Real-world examples demonstrate the effectiveness of no-code AI model builders in various industries. For instance, a retail company could deploy a predictive modeling AI application development to forecast inventory needs. Utilizing tools like Obviously AI, they can automate the data analysis process without any coding.
In another scenario, healthcare organizations use no-code solutions for patient outcome predictions. By integrating models with their electronic health records (EHR), they can enhance service delivery and fine-tune treatment plans based on real-time data.
Overcoming Common Integration Challenges
Despite the many benefits, integration can come with its challenges. Users often face hurdles regarding data access and compatibility issues. It’s vital to address these early by conducting a comprehensive audit of existing data sources and their formats.
Additionally, team communication is essential. Stakeholders must be informed and engaged throughout the integration process to facilitate smoother operations. Regular check-ins can ensure everyone is on the same page.
Using data automation tools can also assist in overcoming these challenges. Implementing automated workflows can streamline the data preparation process, reducing the time spent resourcing clean data for AI model training.
Best Practices for Monitoring and Maintenance
Monitoring and maintenance are crucial to the continued success of deployed AI models. Intermediate users should establish periodic review processes to assess model performance against key metrics. This can help identify any degradation in accuracy over time.
Moreover, having a feedback loop will allow organizations to capture insights from model users and conditions. Implementing these changes proactively can significantly enhance the longevity and reliability of the deployed AI solution.
Finally, continuous training and updating of the models are essential. As the underlying data changes, periodic retraining will ensure that the models remain relevant and accurate in predicting outcomes. By adopting these best practices, businesses can optimize their investment in business optimization tools and ensure effective integration of AI solutions.
Boosting Productivity with BurstyAI
Automating AI Workflow with Ease
With the advent of no-code AI model builders, professionals are now equipped to automate their AI workflows without necessitating extensive coding knowledge. Utilizing platforms like BurstyAI, users can create customized automation flows that seamlessly integrate with existing tools. This removal of technical barriers enhances productivity, allowing users to focus more on strategy and less on implementation.
By adopting no-code solutions, businesses can expedite the AI model creation process. Users can design workflows that automatically process data and deploy models, significantly decreasing the time needed to achieve results. The power of automation not only simplifies repetitive tasks but also minimizes the risk of human error, leading to a more reliable output.
Efficiency in data handling is further enhanced by integrating with popular platforms like Make and Zapier. These integrations allow users to connect various applications, optimizing data flow and communication between tools. In doing so, AI innovation is propelled forward, providing businesses with agile responses to market changes.
SEO Optimization for AI Projects
In the competitive digital landscape, effective SEO optimization is crucial for any AI project. BurstyAI offers intelligent automation tools that facilitate the integration of SEO practices into content creation workflows. By automatically generating keywords and enhancing metadata, users can ensure that their AI applications remain visible in search engine results.
The incorporation of predictive modeling within SEO strategies can also yield impressive outcomes. By analyzing user behavior data and trends, businesses can tailor their content to meet the needs of their target audience, maximizing engagement and conversion rates.
Furthermore, using AI-driven insights, users can continuously refine their strategies, ensuring ongoing relevance in search rankings. This approach positions businesses to adapt swiftly, leveraging data-driven decision-making to optimize their online presence.
Enhancing Content Creation
Effective content creation is central to the success of any AI initiative. BurstyAI simplifies this process through its suite of automated tools that enhance productivity and creativity. By allowing users to create content through a guided process, the platform enables quick adaptation to brand voice, style, and target audience.
In addition, community AI models contribute to enriching content production. These models bring together diverse perspectives and insights, which can be leveraged to produce unique and engaging content. Users gain access to a wealth of knowledge without the need for extensive prior experience in AI.
Partner and Influencer Hunting
Successful AI project execution often hinges on effective partnerships. BurstyAI supports users in identifying and engaging with potential partners and influencers through automated outreach tools. This equips users to broaden their networks intentionally, fostering connections that can enhance their project outcomes.
By offering insights on influencer trends and engagement metrics, BurstyAI allows users to make informed decisions regarding whom to approach for collaboration. With a streamlined process, the time spent on research and outreach diminishes, thus increasing productivity.
Integration with Make and Zapier
Integration capabilities are vital for maximizing the potential of no-code AI tools. BurstyAI’s seamless connectivity with tools like Make and Zapier ensures users can tailor their workflows based on their unique operational needs. This adaptability eliminates bottlenecks, facilitating smoother transitions between different stages of AI application development.
The result is a more efficient project lifecycle, where teams can remain focused on delivering results rather than juggling multiple platforms. With the automation of routine tasks, users can allocate more time to strategic planning and innovation.
Overall, BurstyAI provides a comprehensive solution that enhances productivity across various facets of AI application development and deployment, ensuring that businesses can harness the power of AI without the need for complex coding skills.
Data Management and Ethics
Ensuring Data Privacy and Security
In the world of no code AI model builders, data privacy and security are paramount. With robust tools like Cogniflow and Akkio, practitioners must prioritize secure data management practices. This includes utilizing encryption methods, secure access controls, and regular audits to identify vulnerabilities. A proactive approach to safeguarding sensitive information ensures compliance with legal requirements and builds trust among users.
Furthermore, ongoing training on data security protocols is critical. Leveraging no-code AI tools, teams can automate data processing while maintaining stringent security measures. This balance not only mitigates risks but also enhances the overall efficiency of AI model creation.
Organizations can greatly benefit from implementing cross-functional teams that focus on data privacy. By collaborating, experts from IT, compliance, and data science can offer insights that lead to a comprehensive strategy for data security. Ultimately, a culture of transparency in data handling practices empowers organizations to innovate without compromising security.
Ethical Considerations in AI Model Building
As AI without coding becomes increasingly accessible, ethical considerations emerge at the forefront of AI application development. It is essential for practitioners to contemplate the potential social impacts of their models. This includes examining biases in the data, transparency in methodologies, and the models’ eventual consequences on users and communities.
Implementing ethical guidelines during model building is vital. Teams should establish frameworks that encourage the ethical use of AI innovation while also considering their broader impact. Additionally, community feedback plays a significant role in identifying ethical pitfalls, allowing for more inclusive and equitable models that genuinely serve the intended audience.
The influence of diverse stakeholders can further foster a culture of ethics in AI. Engaging with a broad range of perspectives often leads to richer insights and allows organizations to address concerns proactively, ensuring that their AI solutions reflect a commitment to ethical practices.
Data Governance and Compliance
Data governance forms a critical component of successful no-code AI tools. Organizations must define clear roles and responsibilities for data management. Having a structured governance framework not only complies with regulations but also enhances data quality, making models more reliable and effective.
Furthermore, compliance with regulations such as GDPR and CCPA is non-negotiable. Practitioners must keep abreast of current legislative requirements to ensure their AI initiatives align with legal obligations. Regular compliance checks and engaging legal experts can help mitigate risks associated with data mismanagement.
Continually assessing data governance policies and practices ensures alignment with industry standards and ethical benchmarks. Companies engaging in business optimization tools can leverage governance frameworks that can evolve with the changing landscape of data privacy and AI ethics.
Managing Data Lifecycle Effectively
Managing the data lifecycle effectively is crucial for successful AI model deployment. This involves recognizing the various stages of data, starting from collection to storage, processing, and eventual disposal. By establishing clear protocols for each phase, organizations can ensure data integrity and security throughout.
Leveraging automated workflows in data automation can significantly enhance data lifecycle management. Intermediate users who harness tools like Model.ai can streamline processes, ensuring that data is not only optimized for model training but also archived efficiently when necessary.
Moreover, organizations should adopt strategies that allow for continuous improvement in their data lifecycle management practices. This includes conducting regular evaluations of data usage, and ensuring that data quality remains high over time, which in turn supports effective model outcomes.
Balancing Innovation with Responsibility
Finally, striking the right balance between innovation and responsibility is essential in the realm of machine learning no code solutions. Innovation should not come at the expense of ethical considerations or compliance. By instilling a culture of responsible innovation, organizations can leverage AI model creation to deliver impactful solutions while maintaining trust.
Regular dialogue about the ethical implications of technological advancements can foster a responsible approach to innovation. Engaging with various stakeholders, including those affected by AI outcomes, can help mitigate ethical dilemmas that may arise during the proliferation of no-code solutions.
Furthermore, methodologies that emphasize accountability can reinforce the responsibility dimension of AI development. Organizations that promote ethics alongside innovation are better positioned to achieve sustainable success in this rapidly evolving landscape.
Troubleshooting and Support
Common Issues and Their Solutions
When using no code AI model builders, users may encounter a range of common issues that can hinder their progress. These problems often stem from misunderstandings of the platform’s capabilities or simple misconfigurations. One frequent issue is related to data incompatibility; users may find their datasets not properly formatted for the AI model creation. The solution often involves ensuring that their data adheres to the required specifications, such as appropriate data types and structures.
Another common predicament arises during the AI model deployment phase, where the model may not behave as anticipated. In these cases, it is crucial to revisit the model’s training process. Fine-tuning the model by adjusting hyperparameters or choosing a different algorithm can often produce better results. Users should meticulously review their training data and the methodologies employed to identify potential areas for improvement.
Additionally, users might face slow performance from their AI applications. This can often be attributed to resource limitations, either on their own system or with the chosen platform. To counteract this, scaling up resources or optimizing the data processing pipeline may be necessary to enhance performance.
Leveraging Platform Support Resources
Many leading no-code AI tools such as Cogniflow, Model.ai, and Akkio provide extensive support resources designed to help users troubleshoot issues effectively. These resources often include user manuals, FAQs, and instructional videos that guide users through various functionalities of the platform. By taking advantage of these materials, users can often find answers to their questions without needing to escalate issues.
Furthermore, online support tickets can be a valuable avenue for resolution. Most platforms allow users to submit specific queries directly to technical support teams, who can provide targeted assistance for more complex problems. Basic troubleshooting steps to follow before reaching out can save time and lead to quicker solutions.
Community Forums and Knowledge Sharing
A vibrant community can significantly enhance the AI application development experience. Many no code AI model builders feature community forums where users can share their experiences and insights. These platforms provide valuable opportunities for users to learn from each other, share solutions to common problems, and even collaborate on projects. Engaging with these communities fosters a spirit of collaboration that can lead to innovative solutions and enhanced learning.
Moreover, users can contribute by sharing their own success stories or troubleshooting experiences, further enriching the repository of knowledge available to others. Such knowledge sharing helps maintain a culture of support and continuous improvement within the community, making it a vital component of the no-code landscape.
Continuous Learning and Staying Updated
The field of AI is constantly evolving, and staying updated with the latest advancements and best practices is essential for intermediate users. Subscribing to newsletters, following relevant blogs, and participating in webinars can help users keep abreast of emerging trends and technologies in AI innovation. Many platforms offer regular updates as well, highlighting new features or tools that enhance user experience.
In addition, understanding the implications of new algorithms and models can empower users to make informed decisions about their projects. Continuous learning will not only improve their skills but also boost their confidence in utilizing advanced features of no-code AI tools.
Building a Support Network
Creating a personal network of fellow users can be invaluable in overcoming challenges. Collaborating with peers or professionals who are also navigating the landscape of machine learning no code can lead to fruitful exchanges of ideas and strategies. This support network can consist of local meetups or online groups specifically focused on AI model creation and deployment.
Lastly, actively engaging in these networks allows users to develop relationships that can lead to further opportunities for mentorship and partnership, enhancing their professional growth within the AI space.
Case Studies and Success Stories
Real-Life Examples of AI Success
Numerous companies have successfully leveraged no code AI model builders to enhance their operations. For instance, a retail company utilized Akkio to develop predictive models, forecasting sales trends based on historical data. This approach not only saved time but also reduced the dependency on data scientists, allowing business analysts to focus on actionable insights.
Another example involves a healthcare provider implementing Cogniflow to automate patient appointment scheduling. By creating custom workflows without any coding, they increased patient satisfaction and optimized staff utilization. This illustrates how AI without coding can drive efficiency in critical sectors.
A third scenario includes a marketing firm adopting Obviously AI to analyze customer behavior and preferences. This enabled them to craft personalized marketing strategies, significantly boosting engagement rates. These case studies exemplify how build AI models can streamline various business processes.
Industry-Specific Applications
Across various industries, no-code AI tools are enabling businesses to innovate and optimize. In finance, organizations are employing machine learning no code solutions to detect fraudulent transactions in real time. By automating AI processes, they enhance security while minimizing manual oversight.
The logistics sector is also seeing significant advancements. Companies are using AI model creation platforms to optimize routes and reduce delivery times. This not only improves customer satisfaction but also decreases operational costs.
In education, AI is being utilized for personalized learning experiences. No-code platforms facilitate the development of applications that adapt to the learning styles of individual students, thereby enhancing educational outcomes.
Lessons Learned from Leading AI Projects
Successful AI projects often emphasize the importance of data quality and model validation. Companies that prioritize clean, relevant data tend to achieve better AI innovation. For example, leading AI practitioners now advocate for regular audits of their data sources to ensure continued accuracy and reliability.
Additionally, fostering a culture of collaboration between technical and non-technical teams has proven beneficial. Organizations that facilitate communication and understanding between data scientists and business units tend to execute successful AI application development more smoothly.
Another key takeaway is the significance of user feedback. Leveraging insights from end-users can lead to iterative improvements in AI solutions, ultimately enhancing usability and effectiveness.
Strategies for Replicating Success
To replicate the success of leading AI projects, organizations should focus on scalability. No code solutions allow for rapid deployment and easy adjustments. This scalability ensures solutions can evolve alongside business needs.
Training staff on the use of these platforms is essential. Providing training sessions increases adoption rates and ensures that employees feel confident using AI model deployment tools. This can lead to a more innovative and agile workforce.
Organizations should also consider establishing a dedicated AI team. This team can serve as a central hub for monitoring progress, providing support, and sharing best practices within the company.
Predicting Future Trends and Innovations
The business optimization tools market is shifting towards increasingly sophisticated AI model creation capabilities. Future trends indicate a move towards greater integration of community AI models, where users can share and build upon each other’s work, further democratizing access to AI technology.
Moreover, advancements in natural language processing are expected to make interactions with AI platforms even more intuitive. This will enable users with minimal technical knowledge to engage effectively with powerful AI tools.
Finally, the interest in ethical AI will likely grow, promoting the development of transparent frameworks for AI applications. This supports the broader goal of accessibility in AI while addressing concerns related to bias and fairness in AI solutions.
Summary
In conclusion, the journey to achieving success with a no-code AI model builder is marked by a strategic understanding of various no-code AI tools such as Cogniflow, Model.ai, and Akkio. These platforms provide an accessible pathway to build AI models effortlessly, enabling users to leverage features like data preparation, model training, and deployment without any coding experience. Intermediate users can greatly benefit from recognizing the advantages of these user-friendly AI solutions, which streamline processes and enhance business optimization tools.
Furthermore, as the demand for AI application development grows, the ability to integrate AI innovation into existing systems becomes crucial. By utilizing tools like BurstyAI, users can not only automate AI processes but also enhance their content strategies through SEO optimization and seamless integrations with platforms like Make and Zapier. The emphasis on data automation and ethical AI practices ensures that businesses can engage in responsible and effective predictive modeling as they innovate.
This article embodies the essence of mastering the machine learning no code approach, encouraging practitioners to embrace continuous learning and leverage community resources for ongoing support. As they embark on their AI journey, the insights shared through case studies can guide users in replicating success and adapting to future trends in the ever-evolving landscape of AI.
FAQ
Q: Can you build AI without coding?
A: Yes, it is possible to build AI without coding skills by utilizing user-friendly tools such as Cogniflow, Model.ai, and others geared towards no-code solutions.
Q: Can I build my own AI model?
A: Creating a custom AI model involves several steps including defining objectives, gathering data, selecting suitable algorithms, and testing the model to ensure effectiveness.
Q: Do you need to code to make AI?
A: While traditional AI development typically requires programming skills, no-code AI tools allow users to create AI models without those skills, making the process more accessible.
Q: What are some best practices for data preparation in no-code AI?
A: Best practices for data preparation include cleaning the data, normalizing it, and properly labeling to ensure that the model receives accurate inputs for training.
Q: How can I maximize the capabilities of a no-code AI platform?
A: To maximize capabilities, users should explore advanced features offered by their platform, leverage automation, and utilize platform analytics for better insights and adjustments.
Q: What ethical considerations should be addressed in AI model building?
A: When building AI models, it is crucial to consider data privacy, security, compliance with regulations, and ensuring the ethical treatment of data sources.
Q: How can I troubleshoot common issues in no-code AI platforms?
A: Users can troubleshoot common issues by referring to platform support resources, consulting community forums, and engaging with continuous learning opportunities to keep updated on software changes.
Q: What are some real-life examples of no-code AI success?
A: Numerous case studies illustrate the success of using no-code AI tools in various industries, showcasing how businesses have efficiently integrated AI solutions into their operations.
References
1 – NoCode.ai
2 – Cogniflow
3 – Model.ai
4 – The Complete No-Code AI Guide
5 – 15 No Code AI Model Builder Tools
6 – No-Code AI Model Builder Overview
7 – Obviously AI
8 – Top No-Code Machine Learning Platforms
9 – Best No Code AI Tools
10 – Top No-Code AI Tools of 2024
11 – BurstyAI